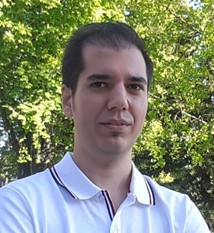
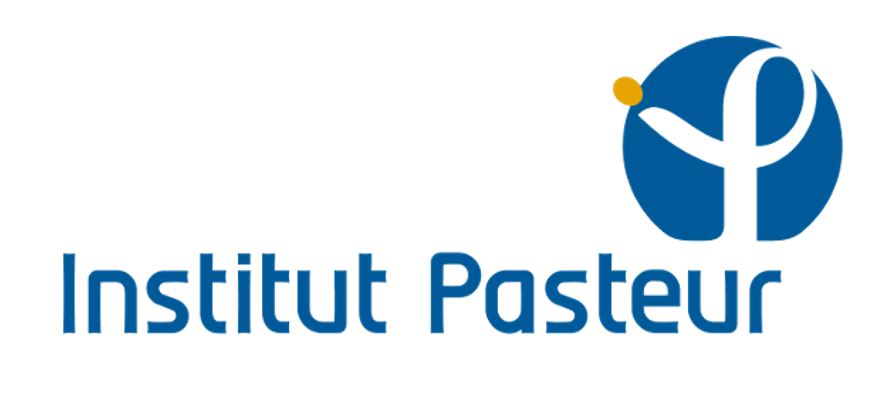
Biography
I hold a B.S. degree in Electrical Engineering and a Master in Biomedical Engineering. During my B.S. I gained experience in engineering, and I developed a particular interest in using engineering methods for the better understanding of living systems. As a result, I continued my education to obtain my M.S. in Biomedical Engineering at Iran University of Science and Technology. My M.S. dissertation was on the diagnosis and treatment of obstructive sleep apnea under supervision of Prof. Erfanian in Iran Neural Technology Center, where has the most equipped laboratories of neural engineering in Iran. Working with Prof. Erfanian and his research team, was a great opportunity for me to develop my knowledge in machine learning and deep learning. In parallel, laboratory experiment offered me a chance to learn about biomedical data analysis.
Host Institution: Institut Pasteur, Paris, France
Superviser: Benno Schwikowski
Secondments
Secondment 1: Integrate different DR analytic techniques.
Secondment 2: Identifying genetic determinants of autoimmune variation
Secondment 3: Conceive and implement a ‘visual analytics’ implementation of the AIMap
ESR 8 Project
Integrated modeling of systemic autoimmune diseases. Systemic autoimmune disease develops as a loss of self-tolerance to ubiquitous autoantigens at the systemic level. The resulting diseases are heterogeneous and vary significantly among patients. Unfortunately, such diseases are so complex that not only no single treatment has shown to be curative, but also the currently available symptomatic treatments may not even result in similar responses among different patients. Rapidly developing technologies to characterize genomic and epigenomic variation across individuals enable personalized “precision” approaches to medicine. Human transcriptome profiles typically contain gene expression values for many thousands of genes, thus representing points in a high-dimensional feature space. A principal technical challenge in characterizing inter-individual variation is to reduce this high dimensionality while preserving biologically relevant inter-individual variation. A second important goal (that may be aligned with the first one) is interpretability of the lower-dimensional variation in the context of other existing biological knowledge, such as molecular pathways or the more general gene interaction networks.
Scientific Interests
- Biomedical signal processing and machine learning
- Cell molecular data analysis
General Interests
- Listening to music
- Watching movies
- Walking and judging
- Novel and scientific books