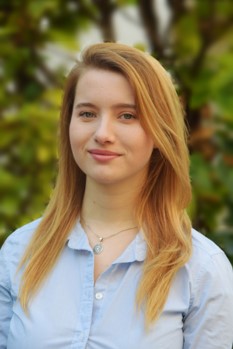
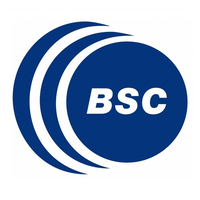
Biography
Upon finishing my Bachelor’s degree in Technical physics I found myself at a crossroads of which path I should choose. I considered pursuing a Master’s degree in either Biomedical engineering or Environmental sciences and eventually decided for the latter as I believed I could apply this knowledge for the benefit of humanity and the planet. I almost continued with a PhD in hydrogeology, but before committing to it, I was inspired to look into other scientific areas, which lead me to come back to my unfulfilled wish of researching biomedical and computer sciences. Although I haven’t exactly followed a traditional and streamlined career path, I consider my versatile knowledge and experiences to be my greatest assets as they provide me with a unique toolbox to solve problems in different and unconventional ways.
Barcelona Supercomputing Center, Barcelona, Spain
Supervisor: Dr. Nataša Pržulj
ORCID ID: https://orcid.org/0000-0002-3262-3275
Secondments
Secondment 1: Validating in vitro predictions (disease genes and potential drug repurposing) through wet lab experiments; Biological analysis of uncovered disease mechanisms
Secondment 2: From validated predictions to medical practice in dermatology using QIAGEN’s “sample to insight” strategies
ESR 9 Project
Patient-centric data integration framework for highly dimensional data
The goal of this project is to develop a patient-centric framework that integrates heterogeneous data types into a single framework (systems-level analysis) to advance precision medicine. The data may include drugs, genes and proteins (multi-omics data), diseases, different exposure chemicals (e.g., environmental molecules, toxins, food & beverage, alternative medicine). Integration will follow a novel non-negative matrix tri-factorization (NMTF) based approach. These types of approaches are characterized by a matrix completion property, which means that the resulting reconstructed matrices contain more information about the system than the original input matrices. Therefore, multi-relational heterogeneous data can be mined to uncover novel relationships between mentioned data types, furthering precision medicine. In addition, NMTF approaches perform co-clustering of the data, which will be utilized for patient subtyping / stratification.
Scientific Interests
- Machine learning applied to biomedical data
- Network science
- Precision medicine
General Interests
- Snorkelling
- Gardening
- Hiking